How will ChatGPT transform the way customers and brands interact?
ChatGPT is causing a stir across the world of business technology. But does reality match the hype? And what do large language models mean for CPaaS and CX?
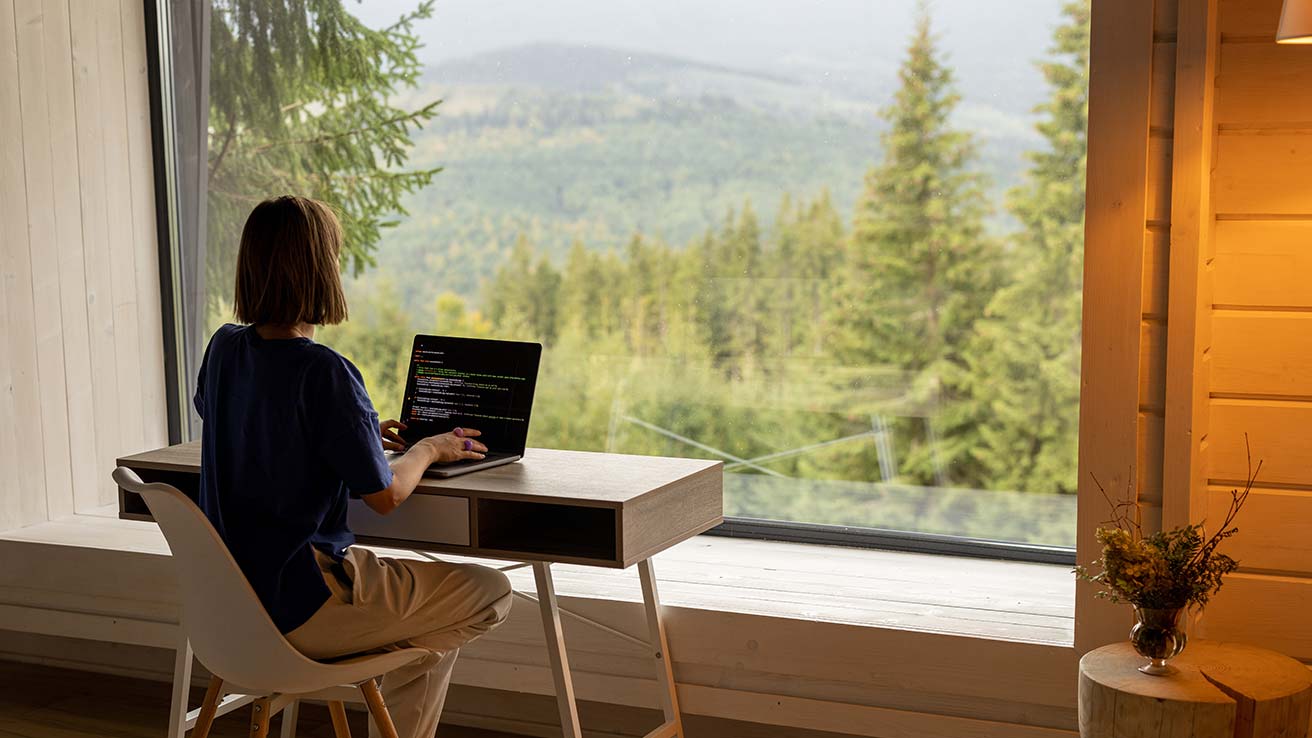
Contents
Large Language Models (LLM) have existed for many years now. And over time, they’ve become increasingly sophisticated and have begun to deliver more lifelike speech and text. But like many technologies, one example has taken the world by storm and made the general public pay attention for the first time. The public launch of ChatGPT is certainly a defining moment for the LLM.
Part of a new wave of pre-trained language models, ChatGPT offers a straightforward interface where users can pose prompts and get back impressively human-sounding text responses. It certainly feels like a cultural moment, partly because of ChatGPT itself and how impressive it is compared to earlier LLMs, but also because of the explosive reaction we’ve seen.
It has already sparked the imagination of millions of people. All kinds of tech and business writers are claiming it will replace our jobs. And The Atlantic’s Breakthroughs of the Year for 2022 even claimed it will transform “how we work, how we think, and what human creativity really is.”
But how much of ChatGPT’s potential is real, and how much is hype? And what does it really mean for the future of work, customer experience, and Communications Platform-as-a-Service (CPaaS) solutions?
ChatGPT is a step forward, but has its limitations
Technology experts have been discussing the rise of LLMs and deep learning for years now. And while some elements of the hype around ChatGPT are well-earned, there are plenty of areas where it doesn’t deliver.
LLMs now have the power to write varied, believable, human-sounding responses to a wide array of prompts. But the technology currently has no real understanding of what it is saying. Even Open AI, the maker of ChatGPT, admits that the tool “sometimes writes plausible-sounding but incorrect or nonsensical answers.”
Likewise, ChatGPT works very well for some tasks but performs poorly in others. It’s fantastic for answering search-like queries, responding to basic writing prompts, and even writing and editing code thanks to its language model being trained on Python code. But when someone asked ChatGPT to write song lyrics in the style of Nick Cave, the singer-songwriter responded curtly saying “this song sucks.” In its current form, ChatGPT won’t give artists, authors and poets much reason to worry.
It’s not LLMs vs. humans, it’s machines and humans together
It’s easy to see the potential of LLMs in replacing human workers that mainly deal in text. But the reality will be that humans and LLMs work together. It’s hard to predict what form this will take and what the result will be, but we can imagine three main changes in the world of work:
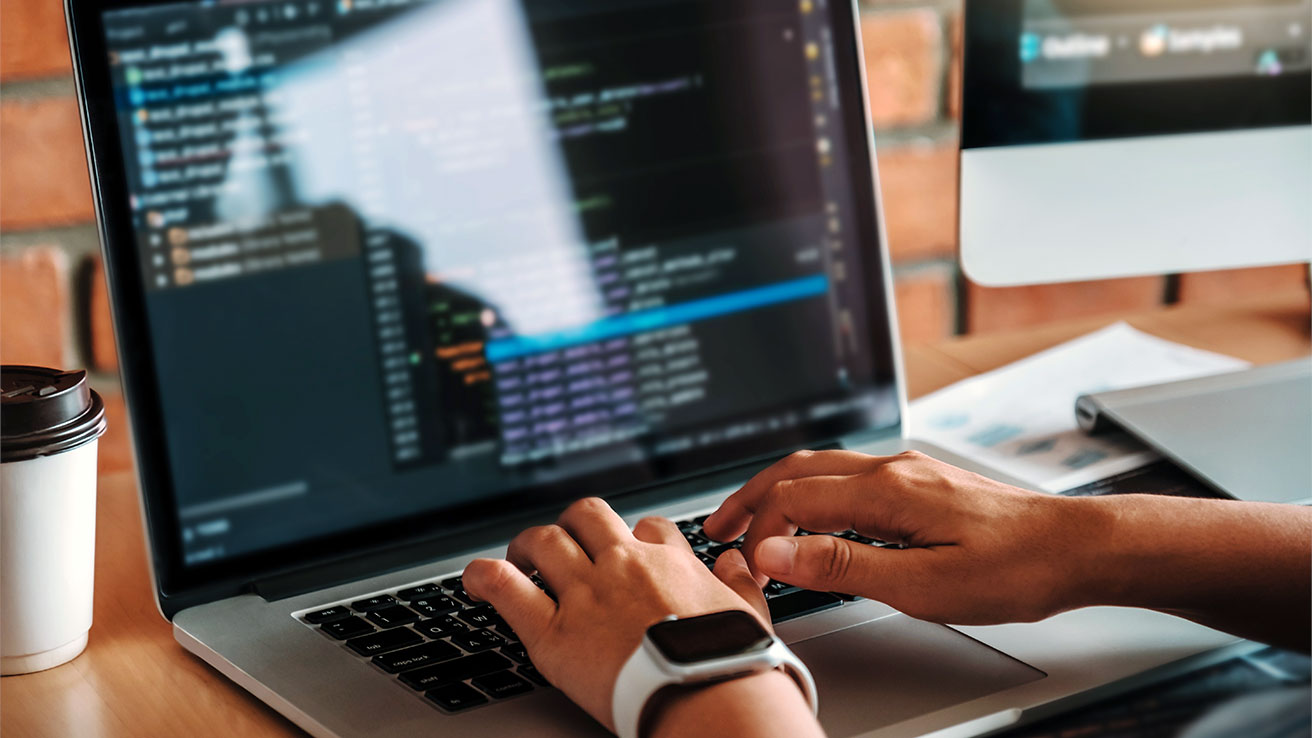
1. Knowledge workers will become more productive
Co-piloting tools will accelerate our capacity to write, edit, create, summarize, and learn. LLMs won’t replace jobs wholesale, but will have a big impact on the nature of employment and the cost of delivering solutions and services. With the ability to easily summarize information, LLMs will help bring new levels of productivity to a broad range of employees.
2. The way we engage with technology will change
From keyboards and websites to intranets and apps, many of the tools we use today are designed to help structure digital inputs and outputs. So, what happens when voice input and digital personal assistants do all those things for you? It’s hard to predict the full impacts LLMs will have here, but they will certainly change the types of technology we use and broaden the pool of people that can access them.
3. The pace of technology change will accelerate
While the ‘wow’ factor of ChatGPT comes from its human-sounding text output, some of the biggest value is in its ability to write code. The way companies work on new digital solutions will be transformed as LLMs learn to write their own software. And developers will be empowered and even more productive with the right AI on their side.
From keyboards and websites to intranets and apps, many of the tools we use today are designed to help structure digital inputs and outputs.
LLMs and CX - the perfect match
While there will be broad changes as LLMs and other generative AI evolve, the impact will be felt particularly keenly in some industries and jobs. We see CX as one of those spaces where the likes of ChatGPT will change the game and influence how CPaaS capabilities evolve. There are three main reasons why LLMs are such a strong fit for the CPaaS space.
CX use cases play to LLMs strengths
Customer-facing chatbots often operate in highly controlled environments where they respond to queries about a set business or type of product. And they frequently work in tandem with human agents and hand over when a customer query is too complex for a bot to handle. ChatGPT for customer support is a perfect fit as LLMs can deliver a great UX, offer human-sounding text to all kinds of questions including questions about products and services, shipping and delivery, billing and payments, and technical support, and quickly summarize information for humans as needed.
The main Achilles heel for the likes of ChatGPT today is offering incorrect answers. But it will be possible to train generative AI technologies to be more accurate when they only have to deal with small pockets of information. While today’s AI will slip up when having to learn a broad spread of topics, there’s no doubt it can memorize your brand’s return policies, for example.
LLMs accelerate trends we already see in CPaaS
We’ve already seen the likes of messaging chatbots bring intelligent automation to the CPaaS space. In that way, technologies like ChatGPT don’t change the game—they merely accelerate progress.
In our recent blog predicting changes in the CPaaS space for 2023, we talked at length about the importance of automation across digital channels—and how this will only become more vital over time. Many CPaaS solutions help brands orchestrate the chatbots we have available today, but when they can integrate with LLMs, the capabilities of these bots will reach all new levels.
But as with today’s bot technology, even the best LLM won’t be able to handle every single circumstance. Some situations will always require the human touch, so brands will still need CPaaS tools that can help deliver a smooth, contextual handover between chatbots and human agents.
Similarly, we also identified personalized content as a trend for the coming year. Whether it’s text or video, LLMs and other generative AI tools can help personalize outbound communications of all kinds at a rapid pace.
LLMs can help CPaaS developers work faster
While plenty of hype has centered around ChatGPT’s ability to write and debug conventional coding languages, it also has plenty of utility in programming workflows, processes, and customer journeys.
Brands will need AI-aware CPaaS solutions that look to the future
While some ChatGPT use cases have some way to go, ChatGPT and other LLMs have come forward in leaps and bounds in the last year. And they’re likely just getting started.
These tools hold huge potential for brands that can successfully integrate them into their contact centers. CPaaS tools can help with this, but only if they have an eye on the future and receive frequent updates to support new LLMs and co-piloting features.
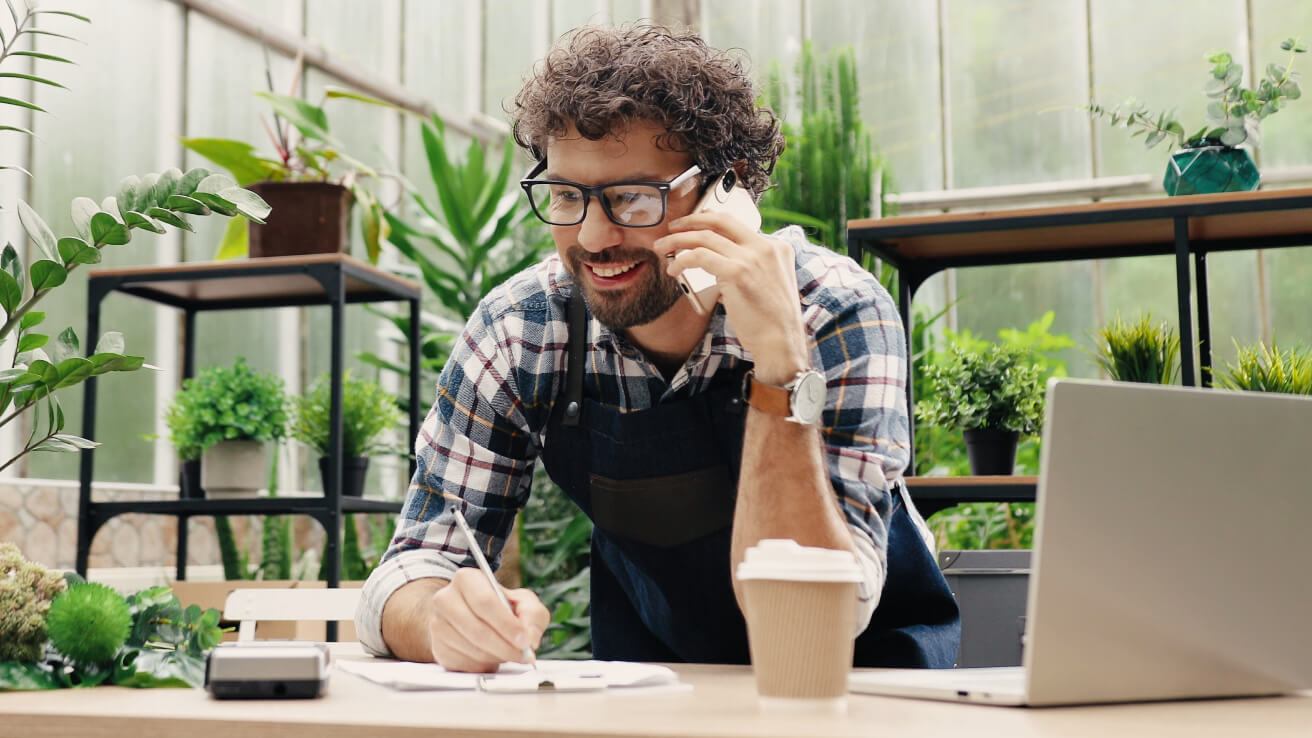
To learn more about how we build chatbot and automation features into our own CPaaS software, and deliver regular updates to keep up with new CX innovations, get the Webex Connect product sheet.
Frequently Asked Questions
-
What is LLM?
Large Language Models (LLMs) are artificial intelligence (AI) models that use deep learning techniques to understand and generate human language. LLMs are trained on massive amounts of text data from a wide variety of sources, including books, articles, and websites, and use this data to learn patterns and relationships in language.
LLMs are designed to be general-purpose language models, meaning they can be applied to a wide range of language-related tasks, including language translation, question-answering, text summarization, and even generating original text. LLMs can generate human-like text that is often difficult to distinguish from text written by a human, and can also be used to understand and respond to human language in a conversational context.
-
What is the difference between machine learning and deep learning?
Machine learning and deep learning are both subfields of artificial intelligence (AI) that involve training algorithms to learn patterns and make predictions based on data. However, there are some key differences between the two:
Complexity: Deep learning is a type of machine learning that involves training algorithms on large and complex datasets using neural networks with multiple layers. This allows deep learning algorithms to learn and represent complex patterns and relationships in the data. In contrast, traditional machine learning algorithms typically rely on simpler models that are not as well-suited for complex tasks.
Feature extraction: In traditional machine learning, features are typically extracted manually from the data and used as inputs to the algorithm. In deep learning, however, features are automatically learned by the neural network during training, which can save time and improve accuracy.
Performance: Deep learning algorithms have shown superior performance in tasks such as image recognition, speech recognition, and natural language processing. However, they typically require large amounts of data and computing power to train effectively.
Interpretability: Traditional machine learning algorithms are often more interpretable, meaning that it is easier to understand how the algorithm is making predictions based on the input data. In contrast, deep learning algorithms can be more opaque, making it difficult to understand how they are arriving at their predictions.
Overall, deep learning is a more complex and powerful form of machine learning that can be used to tackle complex tasks with large and complex datasets, but it also requires more resources and may be less interpretable than traditional machine learning algorithms.
-
How does ChatGPT work?
ChatGPT is a large language model that works using natural language processing (NLP) techniques. It is based on the GPT-3.5 architecture, which means it is a variant of the GPT-3 model, but with some modifications made to improve its performance and efficiency.
The model works by taking in a sequence of words as input and predicting the most likely next word in the sequence, based on its training data. This process is repeated until the model generates a complete response to the user's input.
ChatGPT has been trained on a massive amount of text data from the internet, which allows it to generate responses that are similar to those of a human. The model has also been fine-tuned on specific tasks, such as question-answering and language translation, which further improves its ability to generate accurate and coherent responses.
To use ChatGPT, users can input text-based queries or statements, and the model will generate responses based on its training and fine-tuning. The more information and context provided by the user, the more accurate and relevant the responses will be.
-
What does GPT stand for in Chat GPT?
GPT stands for "Generative Pretrained Transformer". It is a type of language model architecture that uses self-attention mechanisms and pre-training on large amounts of text data to generate high-quality text. The ChatGPT model is based on the GPT architecture and has been further optimized for use in conversational settings.